Data loss prevention (DLP) is an essential aspect of modern cybersecurity. As companies generate and process more data than ever before, the risks of data breaches, leaks, and thefts are higher than ever. Data loss prevention architecture is the foundation … Read More
Cybersecurity
Data Risk, Intelligence and Insider Threats
Data Risk, Intelligence and the Insider Threat When it comes to securing networks in today’s business environment, the single biggest challenge firms must contend with is that of the insider threat. While the term is typically associated with corporate espionage … Read More
Data Security that Works
https://gttb.com/wp-content/uploads/2020/12/Data%20Security%20Works%20GTB_Tech%2090.mp4
Data Security that Works 2021
Data Security that Works 20201
UBA, ITM and Enterprise DLP
User Behavior Analytics, or UBA, is one of the most important developments in digital data protection to emerge in the past several years. UBA is an advanced cybersecurity process designed to detect insider threats, primarily those connected to targeted attacks, … Read More
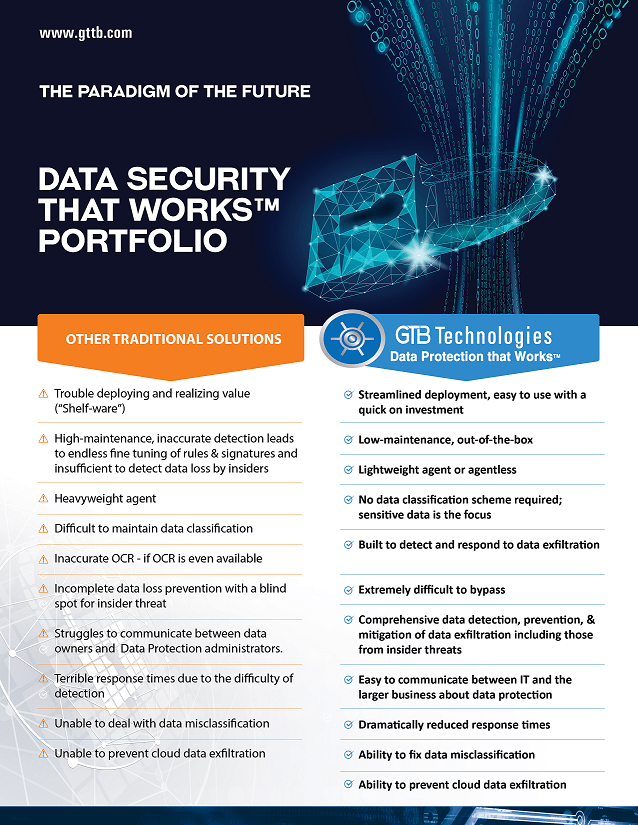
Badmouthing Data Loss Prevention (DLP) is Fashionable
Badmouthing Data Loss Prevention (DLP) is Fashionable Is DLP Really Dead? I recently came across several digital security vendor sites who describe themselves as a “DLP alternative.” Perusing through their pages, I came across comments such as “DLP … Read More